Unlocking LLM Potential: Balancing Privacy and Context Awareness in User Interactions
- Eric Vilanova
- Oct 1, 2023
- 5 min read
Updated: Oct 19, 2023
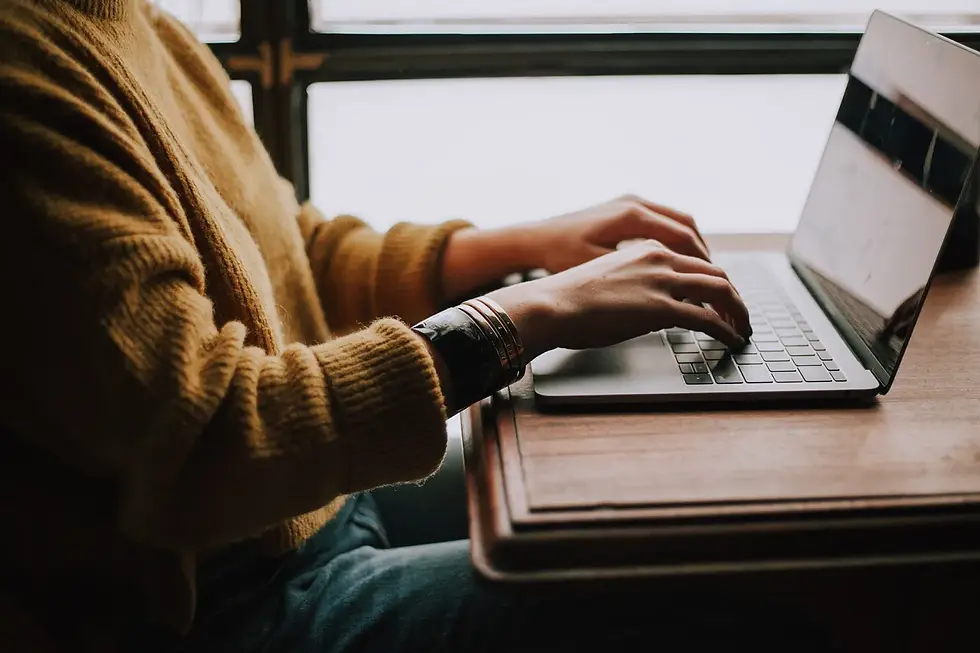
As a Product Designer, it is increasingly important to be able to operate at the critical junction point of design and strategy. One of the most pressing challenges and opportunities in this intersection point lies in strategizing the future state for the next generation of interfaces that will sit atop the plethora of LLMs on the market (Large Language Models). The large majority of the existing products that leverage these LLMs are conversational interfaces in which the user is required to have some prompt engineering ability to extract value and more personalized concepts raise serious concerns about how that individualization is achieved and data privacy. The next generation of these technologies will be what the GUI was to command-line interfaces in early computing. These interfaces should serve to abstract the complexity and empower everyday users to leverage the power of LLMs without having to delve into nuanced prompt engineering.
The Need for Abstraction
LLMs, if you have paid any attention over the past 12 months particularly, have grown incredibly powerful and versatile. They can draft emails, write code, answer complex questions, and even generate creative content. However, to harness this potential, users often require a deep understanding of how to formulate precise prompts and queries. And this precision is not a science. Without a deep understanding of how these models generate information and utilize their sources even among the researchers developing the algorithms, expertise in prompt engineering is often honed through iterative trial and error rather than a precise understanding of the correct methods to consistently achieve the desired outcomes. This creates a significant barrier for the average user who may not possess either the technical or linguistic skills required to interact effectively with LLMs.
Product designers have an opportunity here to bridge this gap by creating interfaces inspired by some of the most unlikely sources that can abstract the complexity of AI models, improve data privacy, and provide more nuanced ways of expressing intent and meaning to LLMs.
1. Learning from Ad Sales Models (With Better Privacy)
With all of the pushback against ad sales models and their data practices of late, this might seem like an odd connection to strive for. However, the UX of LLMs can be significantly enhanced by drawing inspiration from the very customer profiles that have caused much of the dispute while also integrating advanced anonymization and aggregation strategies to mitigate data privacy concerns. By creating customer profiles that leverage aggregated trends and pseudonymization, LLMs can gain valuable insights into user preferences and behaviors without compromising data privacy. This approach ensures that individual user data remains anonymized and secure, while still allowing the model to deliver personalized and contextually relevant responses. By focusing on group profiling and contextual data, LLMs can provide users with tailored content and assistance based on shared interests and immediate interactions, all while respecting user consent and time-limited data retention. The result is a more refined and user-centric LLM experience that strikes a balance between personalization and privacy, ultimately enhancing user satisfaction and trust simultaneously.
2. Context-Aware UI Generation
Context-aware UI generation in LLM interfaces represents a promising avenue to enhance the input/output relationship of LLMs without relying on expert-level prompt engineering. By leveraging this approach, LLMs can intuitively understand user context, needs, and preferences, allowing for more natural and efficient interactions. In this reality, the system would be dynamically adapting the user interface of the input field, presenting relevant options and suggestions for inputs or other interactive elements like sliders and buttons that could better tailor the ongoing conversation or task to the original input. In the image below, I designed a simple chatbot UI which displays an example of this concept. Picture asking your LLM to generate an email for you to send to a coworker requesting their assistance with a task. You might start with a request for an email in a “formal tone”. Rather than the LLM producing an email draft for you and you responding multiple times with suggestions of changes in tone, it might offer up a slider to show where it perceives its initial output lands on a formality scale and you can then more accurately adjust the tone of the email along a formal to informal scale to dial in your intended feel for the message. See Image 1.0 below for reference.

This means that the system would have an improved understanding of the input and users would not need to craft precise prompts to extract their desired information. Instead, the LLM, through contextual awareness, would bridge the gap between the user’s ultimate intent and its output, making the interaction smoother, more intuitive, and ultimately more accessible to a wider range of users, regardless of their expertise in prompt engineering. This innovation has the potential to democratize the use of LLMs and make them more user-friendly, fostering broader adoption across various domains.
3. Context-Aware Anticipatory Assistance
In the spirit of continuing to take inspiration from previous notoriously poor user experiences, another idea here is the concept of anticipatory assistance. While it is easy to think of overzealous autocorrect, intrusive predictive text, and unsolicited help from Microsoft’s Clippy when referencing anticipatory assistance as a feature of LLMs, there are plenty of positive examples of this concept as well. In assessing all of the good examples I could think of, the common denominator underlying all of them was highly specific context awareness. Think of Grammarly’s Chrome Extension. When writing a cover letter or a formal piece of writing, suggestions for changes regarding spelling, punctuation, and sentence structure are welcomed and appreciated. However, this same tool becomes an annoyance when writing an informal note to a friend to make plans. Anticipatory assistance is a great concept but, without contextual awareness, the same tool can be your biggest help and your biggest annoyance when completing tasks. With improved persistence and memory, LLMs can recognize the context you’re operating in and offer suggestions relevant to your current task. If the context is always considered, the suggestions become more helpful and less intrusive. This also does the job of abstracting the chatbot interface of the LLM which requires high-touch interactions and prompt engineering and integrates it into the way we naturally move through our daily lives, switching contexts rapidly and molding our actions to the task that lies in front of us. In the example I designed below, you can see a crude prototype I designed displaying this concept of anticipatory assistance. Based on the LLM’s collection of data from other Figma users, the tool recognizes the kind of project you’re creating and is able to offer design recommendations as you create, using a “copilot” model. This uses anonymized and aggregated data to offer assistance in real-time and in context for the user as they work. View Video 1.0 below for reference.
In summary, the future of AI interaction lies in abstracting the complexity of LLMs through user-friendly interfaces. By creating interfaces that understand and respond to both natural language and more nuanced interactions, LLMs can enable individuals to harness the full potential of AI, without the need for prompt engineering. This disruption has the potential to transform industries, making AI a more integrated and indispensable part of daily operations. As technology continues to advance, the power of AI should be able to be harnessed by everyone, and that starts with the innovative work of product designers.
Comments